Artificial intelligence: Growing fact or lingering fiction
AI adoption in mining and minerals exploration
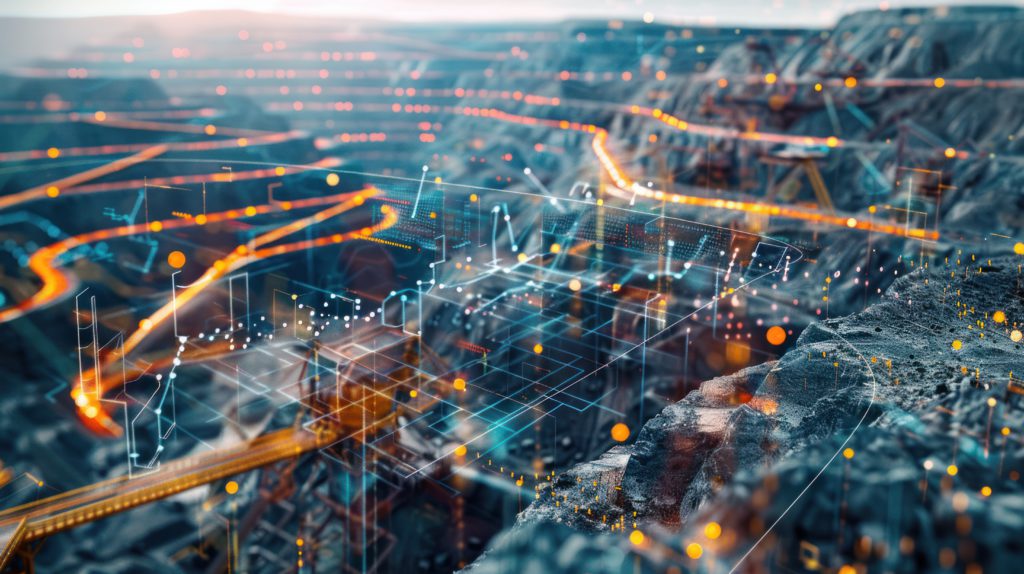
The sales literature is voluminous. Curiosity is still high. But is artificial intelligence (AI) adoption in mining and minerals exploration fully realized or still merely aspirational?
One prominent mining company in Northern Ontario seemed surprised by the question and promised to study it. Another agreed to talk, and surprised me by not having made a single effort towards AI adoption and has no plans to do so. Another who boasted in 2017 about its foray into the digital revolution this year fell strangely silent.
What has made mining and mineral exploration companies suddenly shy about adopting generative AI or even talking about it? Confessed one industry insider, “Much of the investors and players in mining are resistant to these new technologies.”
While Statistics Canada cannot list companies that have taken the plunge into AI or how deeply, it reports a mere 7.3% of Canadian mining enterprise overall invested in AI technology in 2023. Others farther afield remain bullish.
Auckland, U.K.’s Oregon Group, which focusses on investments in critical minerals, mining, and technology cites BHP’s use of AI to automate decision-making with a system that chooses which crusher should be used by trucks to minimize queuing and costs.
Across the Pacific in China, Huawei has launched its intelligent MineHarmony OS platform, using real-time sensors to improve coal productivity. Meantime, Freeport-McMoRan boosted production by 10% at its copper-ore concentrating mill in Bagdad, AZ, after piloting a “transformational” machine learning (ML)/AI model.
No throwing out the baby
Seven years ago, IBM Watson was introduced to ingest, analyze, and synthesize massive amounts of complex geological and survey data at Goldcorp’s Red Lake mine in Ontario. Today, Alex Harvey, senior managing consultant with IBM Canada AI and Analytics was asked how much we are continuing to rely on non-AI methods.
“Generally, teams still search, prepare, and model data and repeat that to spatially review what they are seeing in the field,” Harvey explained. “They still use geostatistical methods like kriging to transfer those field observations like drilling into their wireframe, and interestingly, AI often stems from many of these statistical methods,” Harvey noted. One application of AI has been to synthesize disparate geological data sets to compare say a drill hole log from Argentina with a drill hole log from northern B.C.
The tradeoff: traditional methods tend to be linear, more user-defined, and after “decades and decades of application,” more explainable. “That last task is something IBM continues to place a high premium during AI development,” Harvey stresses. Conversely, AI uses a vast array of relational information to train models to come up with “subsurface characterizations looking at your data in a new way.”

What does a potential client peaking over IBM’s shoulder see once the synthesis of all that geological, geophysical, and geochemical data is complete? In short, answered Harvey, a “heat map” to precisely show the client “here are all your high potential areas” for minerals exploration to make better decisions, increase your hit rate, “and drill fewer duds.”
Another area IBM is making strides in is systems optimization processing at the mining site. “You can optimize each part of a system individually,” Harvey explained. “Whether its crushing, flotation, or concentration, using AI you can scale all of those optimizations across those components,” he concluded.
Forget “big” data?
IBM is not alone on the AI-driven minerals exploration side. In May of this year, VerAI Discoveries was tagged to detect minerals under covered terrain and to minimize surface disturbances at U.S. Critical Materials’ Sheep Creek rare earth properties in Montana.
To fulfill its part of the deal, VerAI begins with programming, not technology. The focus of its “AI Targeting Platform” are those seam deposits below the terrain “that do not have any manifestations on the surface,” explained VerAI’s CEO and cofounder Yair Frastai.

“Instead of sifting through reams of big data, the key is identifying and working the right geophysical data. This is the only data that penetrates the subsurface,” Frastai stressed.
However, VerAI’s platform has also developed a huge library of economic deposits, but it searches for precise patterns of similar geophysical behaviour in new areas it may target for exploration.
“We are not driven by assumptions and subjective opinions of experts,” said Frastai.
VerAI’s approach has enabled it to forge partnerships with companies in Canada, U.S., Peru, and Chile, with more than fifty exploration projects under development. In each of these, the permitting requirements are well-known as are the communities in which VerAI’s AI Targeting Platform is used.
The results have been impressive. Considering that, out of a thousand base metal exploration projects each year, only one will result in the opening of a mine, VerAI’s technology’s ability to find economic deposits that can be mined in substantial quantities is better than one in ten.
A global, industrial standout in AI deployment
Traditional methodologies of mineral exploration like geological mapping, geochemical sampling, geophysical surveys, drilling, and remote sensing “are still a necessary part of mineral exploration,” said Percy Rivera Salas, senior manager, Advanced Analytics at Rio Tinto.
“However, for some years now, Rio Tinto has complemented these traditional methods with AI to improve efficiency and success rates,” Rivera Salas added.
Rivera Salas noted that techniques such as Generative AI/Large Language Models, computer vision, predictive modeling, and digital twins for exploration are becoming increasingly common. Depending on the complexity of the business requirements, Rio Tinto applies different levels of AI/ML technology, including descriptive, diagnostic, predictive, and prescriptive analytics built into its AI solutions.
“Recently, we have extensively applied cutting-edge technologies, such as Generative AI and computer vision, to streamline and automate our operations. These included a knowledge-based chatbot like GPT that extracts key insights from hundreds of documents at various levels,” Rivera Salas added.
Rio Tinto’s pride and joy is its Mine Automation System (MAS), which consolidates data from 98% of its sites to provide operational insights using advanced algorithms. It enables interoperability among diverse autonomous equipment and utilizes AI for tasks like ore body modeling, equipment dispatch optimization, and blast control.
At its main Canadian surface site in Labrador City, Newfoundland and Labrador, the IOC iron ore mine has Minestar and Modular systems installed with active dispatching used for truck decisions. These include “on which loading unit or dump to go to and when to go for fuel,” Rivera Salas noted. Meantime, automated Lumo laser-induced breakdown spectroscopy (LIBS) helps core scanning and rapid determination of mineralization.
Images of core samples are fed into a Generative AI model that uses machine learning and advanced analytics to compare new images with images from historic discoveries. In this way, Rio Tinto can tell if a sample looks like any previously discovered major ore body.
Of growing interest across the industry is the use of AI to prevent collision during deployment of heavy machinery. This past decade, Rio Tinto has actively explored advanced collision avoidance technologies like those deployed in underground mining. Rio Tinto’s current approach includes leveraging extensive machine data, with plans to incorporate AI-driven control over braking and steering to prevent collisions proactively.
“While these initiatives are still developing, our focus remains enhancing AI capabilities to ensure safer and more efficient operations across our sites,” said Rivera Salas.
Weighing the risks and rewards
In 2024, according to a global IBM Institute for Business Value survey, mining faces the same six hard truths confronting more than 2,500 CEOs from more than 30 countries and 26 industries in the generative AI era. Conducted from December 2023 through April 2024, here are just a few of its warnings:
“There is no room for sentimentality,” cautioned the chairperson, Industrial Manufacturing, China. “In the business ecosystem, you must work with the best. Valuing connections over capabilities could be kryptonite for business leaders as they jockey for a competitive edge with generative AI.”

Credit: Rio Tinto.
That does not mean you just roll over when you disagree with your partners. Kazuhiro Nishiyama, president, Kansai Mirai Bank, called it “dangerous if we cannot have heart-to-heart discussions with our partners about how we are positioned to navigate change — and what will happen if things are left as they are.”
Javier Tamargo, CEO, 407 ETR is equally blunt. He added, “People hate progress… many employees see generative AI as something that is happening to them, not a tool that works for them. Business model innovation will require buy-in at all levels of the organization.”
The report concluded, “Generative AI has the potential to shake up the way your business has always worked, driving unprecedented productivity, and revealing new avenues for growth. But those tremors could also crack the foundation — and send everything you have built crashing to the floor.”
Taking AI’s true measure
Is AI adoption in mining and minerals exploration in Canada real or merely aspirational? For his part, Alex Harvey points to a second IBM survey showing enthusiasm for generative AI adoption among Canadian CEOs overall surpasses their global counterparts by 64% to 59%.
For Yair Frastai, the issue is that easy access to mineral deposits is not so easy anymore. Exploration is not performing well and cannot supply what we need at this stage. The main reason is that the low hanging fruit has already been discovered, and there is a great need for new deposits.
Rio Tinto’s Percy Rivera Salas agrees that future geoscientists will have to navigate through a complex, data-saturated environment, with targets and patterns often invisible to the human eye.
The consensus is that ML and data analytics can play an increasingly important role in target generation and resource evaluation. Mining’s future depends on it.
David Godkin is a Canadian freelance mining writer.
Comments