The system that ‘reinvents’ weather forecasting: Model claims to be thousands of times more accurate and faster
The method uses artificial intelligence and learning models based on 80 years of physical data
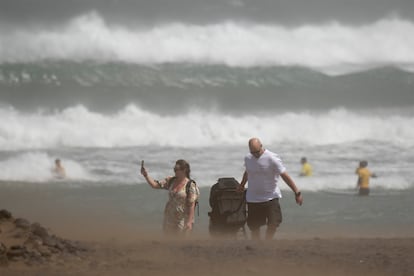
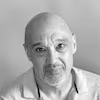
Many people are checking weather apps at the moment to find out what the weather will be like during the Easter holidays, whether the harvest is in danger, what energy windmills and solar panels will generate, whether sailing is allowed, or simply whether they will be able to hang out their washing. Current tools base their predictions on thousands of data points from hundreds of sources that take hours or even days to compile and process using supercomputers. Even so, a forecast of more than three days retains a high degree of uncertainty.
A new system, called Aardvark Weather, developed using artificial intelligence and learning models based on 80 years of physical data, “is thousands of times faster and more accurate than all previous weather prediction methods,” according to Richard Turner of the University of Cambridge’s Department of Engineering and lead author of the research published in Nature.
Turner is excited about the results of the research, carried out by the University of Cambridge with support from the Alan Turing Institute, Microsoft Research, and the European Centre for Medium-Range Weather Forecasts (ECMWF): “Aardvark reinvents current weather prediction methods, offering the potential to make weather forecasting faster, cheaper, more flexible, and more accurate than ever before, helping to transform weather forecasting in both developed and developing countries.”
Numerical weather prediction (NWP) systems date back to the 1950s and are now capable of predicting a wide range of variables up to 15 days in advance. They are based on supercomputer processing of atmospheric models based on decades of recorded observations, fluid dynamics, and statistical post-processing of remote sensing, radar, radiosonde, and aircraft data. The use, development, maintenance, and implementation of these complex systems require significant time and large teams of experts.
Aardvark replaces this entire process with end-to-end machine learning techniques powered by artificial intelligence and, according to its creators, “reduces computational costs, removes bias from some aspects of NWP systems, and enables rapid and accurate prototyping.”
“Using only 10% of the input data from existing systems, Aardvark already outperforms the Global Forecast System (GFS) and is competitive with other weather services that use information from dozens of models and analysis from expert human forecasters,” the researchers say.
And according to Anna Allen, from Cambridge’s Department of Computer Science and Technology and co-author of the paper, “these results are just the beginning.” She adds: “This end-to-end learning approach can be readily applied to other weather forecasting problems, for example, hurricanes, wildfires, and tornadoes. Beyond climate, its applications extend to broader Earth system prediction, including air quality, ocean dynamics, and sea ice forecasting.”
Another application, beyond global and local phenomena, is the development of customized forecasts that, with traditional systems, would take years and cost a huge sum: “Its flexibility and simple design, because it learns directly from the data, can be quickly adapted to produce customized forecasts for specific industries or locations, whether predicting temperatures for African agriculture or wind speeds for a renewable energy company in Europe.”
To defend this claim, Turner specifies: “In just 18 months, we have been able to build something that is competitive with the best of these [conventional] systems, using only one-tenth of the data on a desktop computer.”
Scott Hosking, a research fellow at the Alan Turing Institute, insists that the potential of AI “transforms decision-making for everyone, from policymakers and emergency planners to industries that rely on accurate weather forecasts.”
But like all artificial intelligence models, their results depend on the data they use to develop their proposals and solutions. In this case, the new brain’s memory was provided by the ECMWF ERA5, a combination of eight decades of model data with observations consistent with the laws of physics from around the world. In this regard, Matthew Chantry, strategic director of Machine Learning at ECMWF, highlights the importance of scientific cooperation: “We are delighted to collaborate on this project exploring the next generation of weather forecasting systems. Part of our mission is to develop and deliver operational AI weather forecasts while openly sharing data to benefit science and the wider community.”
“Aardvark represents not only a significant achievement in AI-powered weather prediction, but also reflects the power of collaboration and bringing together the research community to meaningfully improve and apply AI technology,” adds Chris Bishop of Microsoft Research.
Despite the new system, whose creators claim is faster, more accurate, and more efficient than conventional models, weather forecasting still faces the uncertainty generated by the multiplicity of factors involved in highly complex processes.
Emilio Carrizosa, a professor at the Faculty of Mathematics at the University of Seville, who has participated in research on climatic indices of droughts, warns that, in cases like the flash flooding in the Spanish city of Valencia, rare, non-stationary phenomena come into play. “We do not have a sufficient sample to be able to predict the result with certainty. We are talking about phenomena for which we do not have similar or identical data to those we want to study, but only similar ones, and there we may have a bias that we cannot control and that is a determining factor in the phenomenon.”
Dim Coumou, a climate scientist at the University of Amsterdam in the Netherlands, agrees: “Extreme events are, by definition, rare. So you don’t always have many observations. That’s a big obstacle if you want to use artificial intelligence methods.”
Similarly, Argentine astrophysicist Gustavo Romero considers weather prediction one of the most complex processes: “Meteorologists can make probabilistic predictions with a window of, at most, a week. But attempting to do so beyond that is practically impossible because small perturbations in the initial conditions propagate rapidly and produce enormous changes in the results.”
Despite the difficulties, technology giants such as Google and IBM, in collaboration with NASA and other European and international institutions, are working along the same lines as the University of Cambridge with Aardvark, within the framework of the United Nations’ five-year plan: developing artificial intelligence tools that facilitate reliable medium- and long-term predictions and improve early warning systems.
Google DeepMind, the artificial intelligence company of the North American technology giant, demonstrated in the journal Science a machine learning-based weather forecasting model that provides 10-day forecasts that are “better, faster, and more accessible than existing approaches,” according to the study. The model, called GraphCast, outperformed traditional systems in 90% of the cases tested.
The system that Google used as a reference was also the European Centre for Medium-Range Weather Forecasts, which has a supercomputer in Bologna, Italy, with around one million processors and a power of 30 petaflops (30,000 trillion calculations per second). This center, which uses artificial intelligence in its Integrated Forecasting System (AIFS) and offers long-term forecasts of weather events, anticipated the torrential rains in September in Central Europe.
GraphCast, like Aardvark, uses machine learning trained on historical data to provide an accurate 10-day forecast in less than a minute. “We believe this marks a turning point in weather prediction,” say the authors, led by DeepMind scientist Remi Lam.
IBM is also in this race, collaborating with NASA, with a machine learning proposal. “Foundational AI models that use geospatial data [from weather, sensors, and satellites] can be game-changers because they allow us to better understand, prepare for, and address the many climate-related phenomena that impact the health of our planet in ways and at speeds never before seen,” explains Alessandro Curioni, vice president of Accelerated Discovery at IBM.
Sign up for our weekly newsletter to get more English-language news coverage from EL PAÍS USA Edition
Tu suscripción se está usando en otro dispositivo
¿Quieres añadir otro usuario a tu suscripción?
Si continúas leyendo en este dispositivo, no se podrá leer en el otro.
FlechaTu suscripción se está usando en otro dispositivo y solo puedes acceder a EL PAÍS desde un dispositivo a la vez.
Si quieres compartir tu cuenta, cambia tu suscripción a la modalidad Premium, así podrás añadir otro usuario. Cada uno accederá con su propia cuenta de email, lo que os permitirá personalizar vuestra experiencia en EL PAÍS.
¿Tienes una suscripción de empresa? Accede aquí para contratar más cuentas.
En el caso de no saber quién está usando tu cuenta, te recomendamos cambiar tu contraseña aquí.
Si decides continuar compartiendo tu cuenta, este mensaje se mostrará en tu dispositivo y en el de la otra persona que está usando tu cuenta de forma indefinida, afectando a tu experiencia de lectura. Puedes consultar aquí los términos y condiciones de la suscripción digital.